“In the beginning was the Word, and the Word was with Psychology, and the Word was Psychology” ~New Vector Translation
All personality constructs are first described by words. From Freud’s cocaine-fueled models to the staid Big Five, they are all at one point words. Much of academic psychology is concerned with comparing constructs. To do this, they must share a basis, typically a set of subjects. Subjects are given an instrument (usually a survey) which approximates where they live in construct space. Based on how subject responses covary, general claims are then made about the constructs. In this post we explore another way. Advances in NLP allow quantitative comparison of constructs in their natural habitat: language.
Roadmap
In the last post I argued that the Big Five are word vectors. This post makes the same claim about standalone scales, which then allows constructs to be compared without involving subjects. To demonstrate this, a broad personality model is introduced, as well as a method to represent constructs in word space. The outline follows:
Compare constructs in subject vs word space
Problems with subject space
Relate kin and reciprocal altruism to the Big Five using subjects
The same comparison in word space
Introduce a (temporary) five factor model identified using NLP
Project altruism words into that space
Code available here.
Discussion, limitations, future work
A tortuous path
To compare altruism with the Big Five, signal must travel through many transformations: Altruism (ideal) → described by words → survey developed (and hopefully validated) to approximate this construct → administered to subject who interprets these words and searches their soul → subject altruism score → correlation in subject space ← subject Big Five Inventory (BFI) score ← subject interprets BFI items and searches soul ← develop BFI to approximately measure this ← construct defined/communicated by qualitative description ← Big Five (defined by word loadings). Claims are then made about the two ideals, Altruism and the Big Five.
The straight and narrow
Why not use word vectors as the shared basis instead of subjects? The path is far more direct: Altruism (ideal) → described by words → vectorized to word space → comparison in word space ← Big Five, which already exist in word space, as discussed in the previous post. For those keeping score, that’s 4 vs 10 transformations. (It’s scored like golf.)
Tal the Revelator
The difficulty of using subjects to make claims about verbal constructs is not secret.
“Most theories and hypotheses in psychology are verbal in nature, yet their evaluation overwhelmingly relies on inferential statistical procedures. The validity of the move from qualitative to quantitative analysis depends on the verbal and statistical expressions of a hypothesis being closely aligned – that is, that the two must refer to roughly the same set of hypothetical observations. Here, I argue that many applications of statistical inference in psychology fail to meet this basic condition.” ~Tal Yarkoni, The generalizeability crisis
Validity here refers to a score capturing the construct it is intended to measure. It’s worth reading the arguments and examples in full. But for us the take home is what can be done, given these realities. He suggests:
Do something else (enter other fields).
Embrace qualitative research
Adopt better standards (including 7 suggestions).
“One is always free to pretend that small p-values obtained from extremely narrow statistical operationalizations can provide an adequate basis for sweeping verbal inferences about complex psychological constructs. But no one else— not one’s peers, not one’s funders, not the public, and certainly not the long-term scientific record—is obligated to honor the charade.”
Even if your view of research in psychology is not so dim, surely readers have been burned by papers that make claims but employ experiments that are only tenuously related. All available solutions are painful. The field may have to adopt a narrower view and leave the big questions to those studying history, literature, and linear algebra. I propose another way forward.
Convert to wordspace
. 4. Use word vectors as a shared basis
Constructs live together in word space, and yet when comparisons are made we drag them into subject space. This is an enormous, lossy, hassle. What if they could remain safely in word space? The conceit of natural language processing is that words are vectors in continuous space. Analyzing these vectors works well enough to be a load bearing process in trillion dollar industries, and is currently being (re)introduced to psychology.
Practice what you preach
Here we will look at a traditional study done in subject space then try to improve it by moving to word space. Hedging against a straw man, the object is Kin Altruism, Reciprocal Altruism, and the Big Five Personality Factors which has been cited hundreds of times and whose first author has an h-index of 70.
Subjects are measured using three instruments: Big Five (via a survey of adjectives), Empathy/Attachment and Forgiveness/Non-Retaliation (survey of phrases), and altruism in a money-divvying game. Because the authors hypothesize that the interstitial space between Agreeableness and Emotional Stability (AKA Neuroticism) differentiates the two altruisms, some words are added to better measure that area. Similarly, a new questionnaire is designed to measure Empathy/Attachment and Forgiveness/Non-Retaliation, which are theorized as related to kin vs reciprocal altruism respectively. Above and beyond for this type of study, a game is used to measure altruism.
“In the version of the money allocation task used to measure kin altruism, the other person was described as a close friend—someone with whom the participant had a long history of friendship and with whom the participant had much in common. We hoped that by describing the friendship as an old one and the friend as someone very similar to the participant, the friendship would closely resemble the relationship one has with a relative. The reason why we did not use a relative as the potential object of altruism was to avoid introducing variance in responses due to the particular relative involved; for example, many people might be more willing to behave altruistically toward one sibling than another.”
In other words, as to not sully the data with real world feelings towards kin, reciprocal altruism is measured.
“In the version of the money allocation task used to measure reciprocal altruism, the other person was described as a non-cooperator—someone who had been rude, nasty, and inconsiderate toward the participant.”
And to measure reciprocal altruism they measure … non-reciprocated altruism? Naturally there are correlations and the authors conclude:
“The results of this study support the suggestion that personality traits involving empathy and attachment facilitate altruism that is primarily directed toward kin (i.e., kin altruism), and that personality traits involving forgiveness and non-retaliation facilitate altruism that is primarily directed toward non-kin (i.e., reciprocal altruism).”
But if reciprocal altruism was never measured, how can the results support that claim?Statistics in psychology papers are, as Tal points out, often a rhetorical flourish. But we don’t have to play along! Let’s see what word space has to say.
A land of milk and honey (welcome to word space)
In traditional studies, due to costs of mapping subjects onto personality space, resolution can only be high in a few personality areas at a time. That is why the authors probed Empathy and Non-Retaliation and the space between Agreeableness and Emotional Stability. All of those axes exist in regular Big Five space, but are measured quite granularly. In word space we can compare altruism to the complete Big Five in all their hi-res glory. On my github there are 2819 word vectors factorized down to five PCs. We can use those for convenience. The first order of business is to select word sets that describe each altruism. For kin words I chose those that embody familial roles: brotherly, sisterly, mothering, motherly, fatherly, grandmotherly, grandfatherly, wifely, maternal, paternal. For reciprocal altruism, I follow Trivers’ definition.
“Regarding human reciprocal altruism, it is shown that the details of the psychological system that regulates this altruism can be explained by the model. Specifically, friendship, dislike, moralistic aggression, gratitude, sympathy, trust, suspicion, trustworthiness, aspects of guilt, and some forms of dishonesty and hypocrisy can be explained as important adaptations to regulate the altruistic system. Each individual human is seen as possessing altruistic and cheating tendencies, the expression of which is sensitive to developmental variables that were selected to set the tendencies at a balance appropriate to the local social and ecological environment.” The Evolution of Reciprocal Altruism, Robert Trivers (bolding added)
Considering this, I chose: discriminating, unforgiving, vengeful, loyal, neighborly, cooperative, trustworthy, and fair. This is about equal on erring towards cooperation but following up with moralistic aggression when things go wrong. Additionally, it tries to capture this altruism as the antithesis of cheating (eg. fair, trustworthy).
(For an excellent explanation of the evolution of trust, see this interactive demo.)
May I introduce you to the unknown Five Factor Model?
Theoretically, we could use Big Five word loadings produced via surveys, but most of these words are rare enough to not be included. This is for the best as one would not get a good estimate of grandmotherly by self-report among psychology students. As such, word vectors computed using the language model RoBERTa. Derived from a large and well-characterized list of personality words, the resulting Five factors are, in brief:
Affiliation (or Socialization). How much do you want this person on your team? Similar to Agreeableness but precludes being a doormat. Gullible, for example, loads neutrally on Affiliation but positively on Agreeableness.
Dynamism. Quite a close match to Extraversion, but more about a sense of adventure, and less about confidence.
Order. Conscientiousness with an edge. Ability to achieve your own goals. Exacting vs mushy.
Emotional Attachment. Whereas Neuroticism is concerned with instability, this is about attachment; both caring and spiteful are highly loaded.
Transcendence. This factor is characterized by unique, complicated, star-crossed, handicapped, mystical, heartbroken, other-worldly vs. unphilosophical, fancy-free, pigheaded, boorish, materialistic, self-centered, glib. It involves looking beyond oneself and the mundane. That process is, apparently, wound up in pain. In fact, "troubled" loads more on Transcendent than Emotional Attachment (the factor related to Neuroticism).
The names for the first three factors are lifted from De Raad’s pan cultural work because, qualitatively, the match is closer than with the Big Five. Each factor deserves its own post. (For those in industrial psychology, I suspect that Order is more correlated with business success than Conscientiousness as it’s more calculating than showing up on time.) But proposing models is not my forte, and finer language studies are forthcoming which may yield different structure. For now these factors suffice. Here is their correlation with the Big Five:
Results
Altruism word loadings on the first four factors (Transcendence is not important in this study):
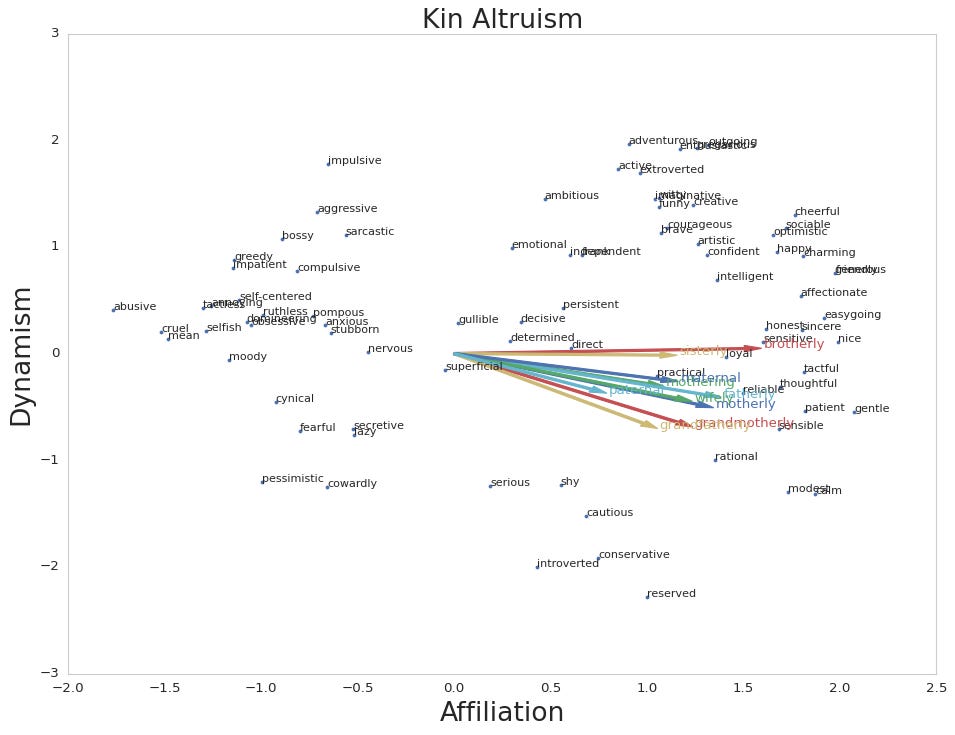
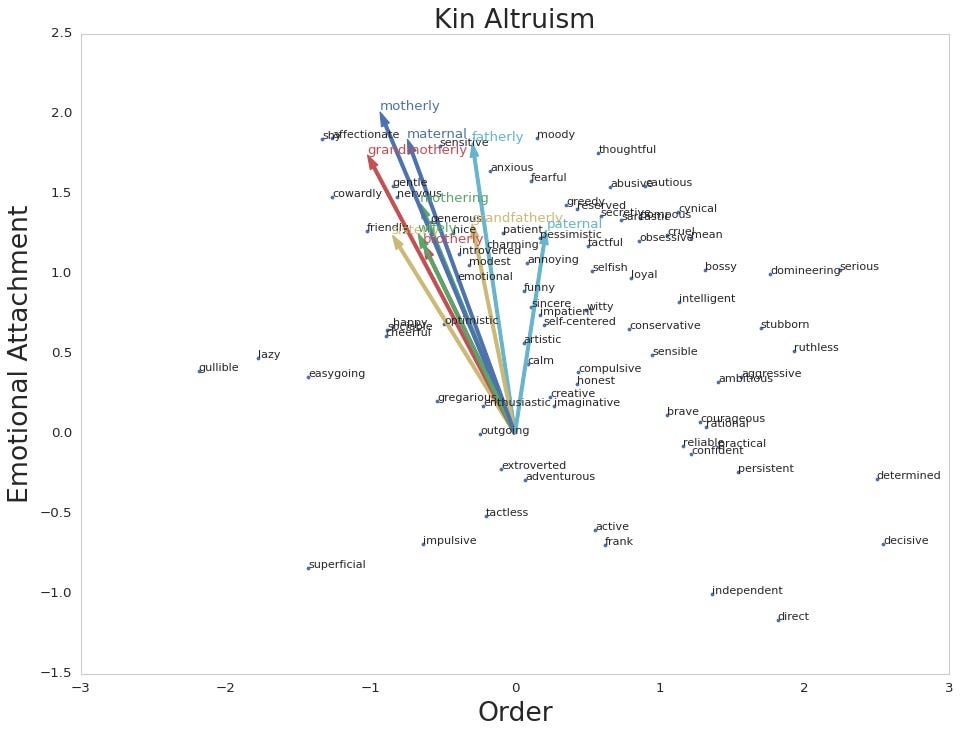
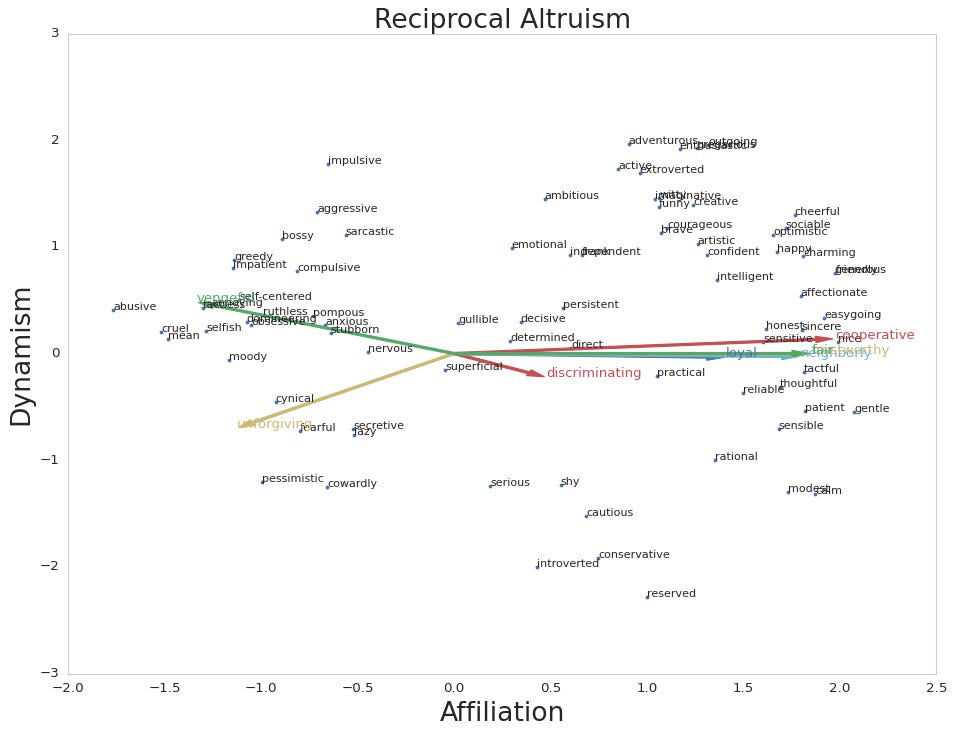
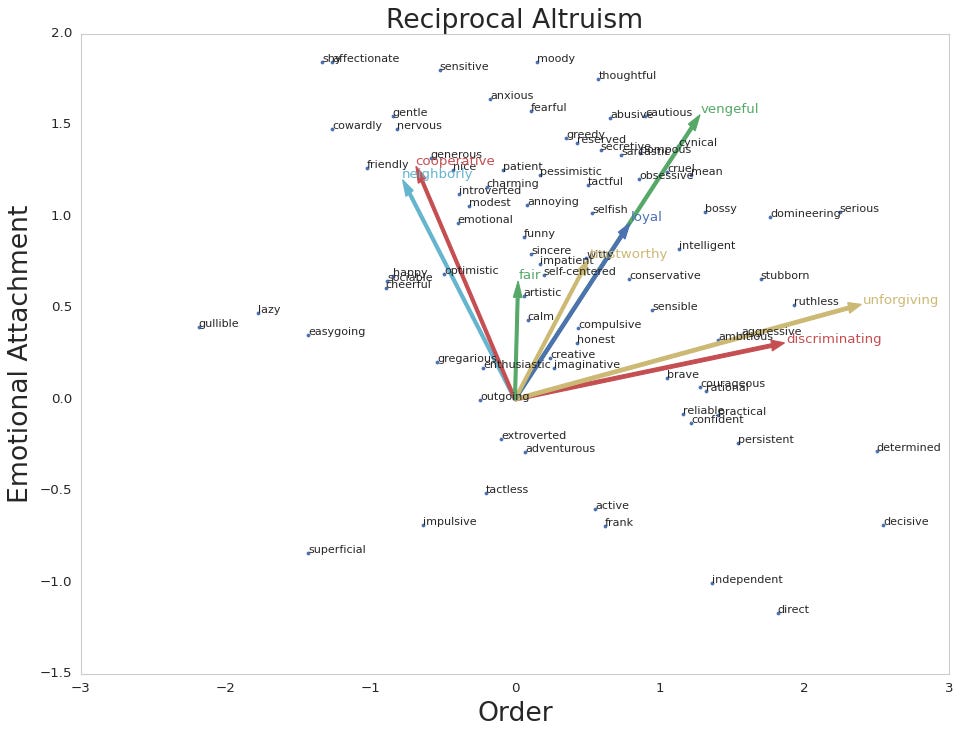
To compare the altruisms, we would like to collapse each of these word sets down to just one vector. (There is room for debate if that even makes sense given reciprocal—and to a lesser extent kin—requires different responses to different scenarios.) The cheap and dirty solution is to treat each construct as a Bag of Words and take the average. The mean loadings are:
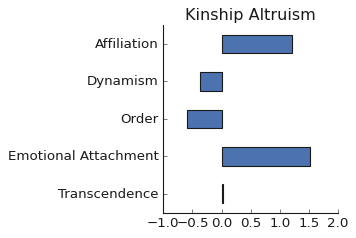
Discussion
I don’t think the paper in subject space includes a valid measure of kin or reciprocal altruism and as such doesn’t add to our understanding of how it relates to personality. This is a surprisingly common failure mode. Word space provides some insurance against invalid comparisons. We have better intuition for where a word should be in word space, than for how subject 112 should answer a survey. It’s easier to spot errors.
From a Bayesian point of view, something different is happening in subject vs word space. Experiments including subjects seek to bring new information to the table. The hope is that will update readers’ view of the world. But researchers (and lay folk) have lots of social experience and more acute perception of psychological processes than the snapshot provided by a survey. It’s hard to move the needle much. Word space is more akin to visualizing our priors than producing new knowledge. This view is valuable because language is where the rubber meets the road, so to speak. As JL Austin put it:
“Our common stock of words embodies all the distinctions men have found worth drawing, and the connections they have found worth marking, in the lifetime of many generations: These surely are likely to be more numerous, more sound, since they have stood up to the long test of survival of the fittest, and more subtle, at least in all ordinary and reasonable practical matters, than any that you or I are likely to think up in our armchair of an afternoon—the most favorite alternative method.” A plea for excuses
Analysis in word space is comparatively straightforward. Rather than tables of correlations and p-values, words are simply plotted on the axes of interest and visual judgements are made. Kin altruism words cluster tightly on both Affiliation and Emotional Attachment, the only two factors with considerable loadings. Fathers, but not brothers, are less attached than their female counterparts, in line with Trivers’ theory of parental investment. Brothers and sisters have as much reason to look after their siblings. Fathers, however, have less reason than mothers. Sperm is cheap. Eggs and pregnancy are expensive.
Reciprocal words are more spread out, reflecting traits ideal for responding to different scenarios: partner cooperation or defection. The most salient difference is higher average loading on Order—accomplishing one’s own goals. Initially called delayed return altruism, reciprocal altruism is not about sacrificing oneself for a stranger but rather investing in your own future through pro-social means. On the other hand, kin altruism refers to helping family even at your own expense because of the selfish genes tugging at your heart strings. This is apparent in higher loadings of kin altruism words on Emotional Attachment, supporting Ashton’s hypothesis. But the main action is on Order, far from where the subject-space instruments were designed to detect. The costs of sampling in subject space make results more dependent on researcher priors.
Interpreting these plots can feel like reading tea leaves, but I’m about 70% sure of what’s here. One thing that gives me pause is that the two altruisms are represented in different ways. Kin words all describe relationships (eg. mother, father, brother), while reciprocal words are a mix of relationships (eg. neighbor) and traits fit in repeated positive-sum games (eg. vengeful, discriminating, cooperative). Uncertainty aside, wouldn’t it be cool if in an afternoon I ran an experiment that combines the lived altruism experience of millions of people? What generations have agreed makes someone paternal, sisterly, or neighborly. As always with a new source of signal, one starts shooting from the hip. Eventually the Wild West is tamed; methods and heuristics emerge. There is much room for improvement. Readers can tweak the word sets and get new results in a matter of minutes using this colab notebook. Please do so!
Advantages of word space
Connected to the Lexical Hypothesis. Grounded in decentralized social reality.
Fewer transformations. Each step is lossy and introduces bias.
Less statistically intensive after the conversion to word space. (Lower barrier to entry.)
Effective sample size (those that contributed to the language model via comments on reddit, writing books, or pubMed articles) is much larger and diverse than most studies.
Better job prospects for psych PhDs who know NLP.
Easier to do multilingual/multicultural work. Some models are trained on 100 languages simultaneously (which is how Meta trains hate speech filters in languages with few examples).
Open science.
Disadvantages
More moving parts. There are billions of parameters in a language model! However, even billions of neurons and dozens of training decisions can result in a stable representation. Any language model worth its salt can complete the analogy “husband is to wife as king is to ____”.
Can’t break results down by demographic. Sometimes it’s interesting to know the personality of elementary school teachers between 25-30. Or to know how some construct correlates with arrest record. Impossible in word space.
Aren’t language models biased? Well, not more than self-report.
Defining altruism as the sum of a bunch of word vectors (ie, a bag of words) is a bit hacky. There is substantial room for improvement here.
Foreign gods
“I think Kafka was right when he said that, for a modern man, state bureaucracy is the only remaining contact with the dimension of the divine.” ~Zizek, A Pervert’s Guide to Ideology
He’s describing here, of course, the transcendent feeling of filing an appeal with the IRB. I have a prediction. Word space is the good and right thing to do from a signal processing perspective, but its adoption will be driven as much by the convenience of not being regulated. The corollary is that the IRB will be the first government body to declare language models sentient.
Preparing the way
We would like to extract relationships between constructs from language models. To do so in a way that adds signal rather than more noise requires a lot of validation work. Initially, this means comparing to well established survey results. Can they be recovered using word vectors? Where do they fail? Once that has been established, every paper that ends “more research needed” should find a way to ask the question in word space.
I spent more than a year fine-tuning a method to extract personality relationships from RoBERTa, the state of the art model at the time. Soon after GPT-3 was released and it performed better right off the shelf. That compute supersedes domain knowledge is a reoccurring bitter lesson within AI. Compute increases exponentially. If you can get 30% gains over a general ML solution using domain knowledge, you can also just wait for compute to catch up and get the same results using general methods. Finding ways to relate psychology questions to off the shelf NLP models is therefore a good way forward. A new model with noticeably better performance is made public every six months or so. Those validating word space are preparing the way for greater intelligences—PaLM, GPT-7, OSCar (Optimal Sentience Cartography)—to rain down psychological truths.
Natural language is teeming with shared theories about the world. Now that we can quantify them, can’t they be used as evidence?
"However, even billions of neurons and dozens of training decisions can result in a stable representation. Any language model worth its salt can complete the analogy “husband is to wife as king is to ____”."
Beware that this isn't really the case: https://blog.esciencecenter.nl/king-man-woman-king-9a7fd2935a85